Sella - Modeling Self-evolving Collaborative LLM-based Agents
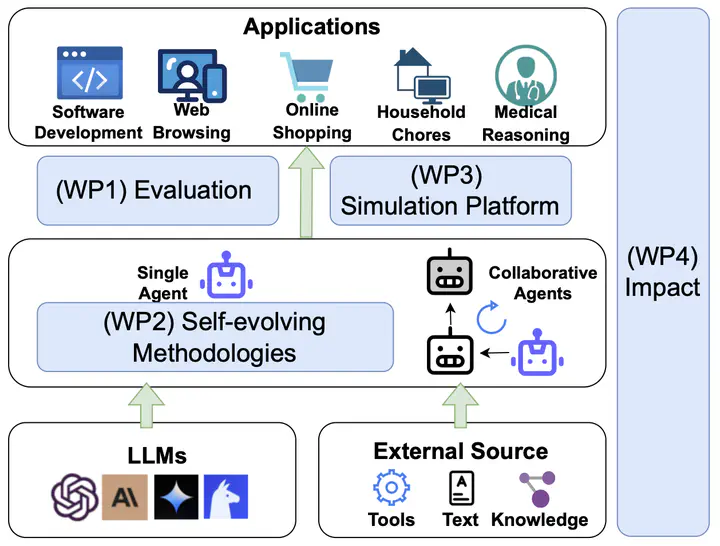
Sella: Modeling Self-evolving Collaborative LLM-based Agents
This proposal was originally submitted to the 2024 Cooperative AI Foundation (CAIF) and UKRI FLF, but was not selected for funding. However, we remain committed to bringing this project to life. Check out my Google Scholar for my latest research related to this project.
Vision
Background
Recent advancements in artificial intelligence (AI) have been significantly driven by the development of large language models (LLMs) such as GPT-4 and their effectiveness in various tasks, including dialogue generation, machine translation, question answering, and other open-ended generation tasks. LLM-based agents are AI systems that leverage the powerful language understanding capabilities of LLMs to interact with the world, make decisions, and perform tasks autonomously.
Building generally capable LLM-based agents is an active area for both academia and industry, aiming to create artificial general intelligence (AGI) systems that can handle a wide range of tasks through reasoning, planning, and tool use. By assigning different roles to multiple LLMs, LLM-based agents can form a collaborative entity for solving complex tasks, such as software development and medical diagnosis/reasoning. Several proof-of-concept demos, such as AutoGPT, LangChain, ChatDev, and MetaGPT, are inspiring examples under this vision.
Currently, single-agent systems can perform tasks such as web browsing, online shopping, and household chores. However, as task complexity increases, the limitations of single agents become apparent, particularly in handling multifaceted environments. For instance, a software development task typically involves multiple stages, including requirements gathering, design, coding, testing, deployment, and maintenance.
To address this complexity, humans have developed Standardized Operating Procedures (SOPs) across various domains, widely used to solve complex tasks collaboratively. The intricacy of these processes highlights the need for more sophisticated collaborative AI agent systems to effectively navigate and contribute to such multifaceted projects.
Collaborative LLM-based agents, which involve multiple LLM-based agents working together to solve problems, present a promising frontier. This collaboration can take various forms, such as task delegation, information sharing, and mutual reinforcement. The collective intelligence of these agents has the potential to surpass the capabilities of individual agents, leading to more robust and versatile AI systems. Recent studies have demonstrated that multi-agent collaborations yield more effective solutions for tasks requiring collaboration and communication efforts, such as software engineering and medical reasoning. By automating complex tasks across various sectors, collaborative LLM-based agents enhance efficiency and effectiveness, providing adaptable and robust solutions to meet the growing demands of modern AI applications.
Research Problem and Hypothesis
The fundamental knowledge gap addressed by this project is: “How can we enable collaborative LLM-based agents to improve their effectiveness in solving complex tasks through self-evolution?” This central research question is broken down into two key hypotheses:
- H1: The effectiveness of collaborative LLM-based agents can be efficiently assessed by automating evaluators consisting solely of multiple LLM-based agents.
- H2: The effectiveness of collaborative LLM-based agents can be enhanced through self-evolution, facilitated by the mutations of individual agents and the optimized structuring of multiple agents.
Research Objectives
To address these limitations, this project aims to develop a package of methodologies for evaluating and modeling self-evolving collaborative LLM-based agents. The project has the following three objectives:
- O1: Automating LLM agents evaluation with collaborative LLM agents.
- O2: Research and develop new methodologies that model the self-evolution of single agents and collaborative agents.
- O3: Developing a versatile simulation platform for the self-evolution of collaborative LLM agents.
Approach
Work Packages (WPs)
To deliver upon the three project objectives, the project is broken into four work packages (WPs):
- WP1: Automating LLM-based Agents Evaluation via Agentic Evaluator (Months 1-6)
- WP2: Research and Develop New Methodologies for Agent Self-evolution (Months 7-12)
- WP3: Developing a Versatile Simulation Platform for the Self-Evolution of Collaborative LLM-based Agents (Months 12-18)
- WP4: Public Engagement and Impact Maximization (Months 7-18)
Project Timeline and Management
The project’s work plan consists of four work packages distributed across an 18-month timeline:
Quarter | WP1 | WP2 | WP3 | WP4 |
---|---|---|---|---|
Q1-Q2 (2025) | ✅ | |||
Q3-Q4 (2025) | ✅ | ✅ | ✅ | |
Q1-Q2 (2026) | ✅ | ✅ |
Recruitment for an 18-month PDRA will commence three months before the project begins. WP1 will extend over approximately 6 months, WP2 will span another six months, and WP3 will commence midway through WP2 and cover the remaining duration of the project. WP4, focusing on maximizing the project’s impact, will begin in the sixth month and continue with periodic activities throughout the project’s duration.
Significance of the Research
This project aims to deliver 3-4 research papers to top-tier conferences such as NeurIPS, ICLR, EMNLP, and ACL. We will be making the codes, models, and platforms publicly available, ensuring accessibility for researchers and practitioners worldwide.
The Sella project will have profound social significance, particularly in enhancing medical reasoning and advancing sustainable AI development. By leveraging self-evolving collaborative LLM-based agents, the project has the potential to revolutionize healthcare and improve various AI-driven processes.
Risks and Mitigations
- WP1 Risk: Metrics may not fully capture all aspects of LLM-based agents’ performance. Mitigation: Iterative testing and feedback loops.
- WP2 Risk: Complexity in dynamically adjusting communication weights between agents. Mitigation: Leverage expertise in dynamic graphs and graph semantics.
- WP3 Risk: Simulation platform may not cover all necessary scenarios. Mitigation: Incremental development with early feedback integration.
- WP4 Risk: Insufficient research outcomes impacting deliverables. Mitigation: Leverage PI’s experience in NLP and AI research.
Pathways to Impact
- Target publications in top AI venues (NeurIPS, ICML, ICLR, ACL, EMNLP, NAACL).
- Open-source all research outcomes, including models, data, and simulation platforms.
- Collaborate with academia and industry (e.g., LangChain, ChatDev, MetaGPT).
- Organize workshops and conferences to disseminate findings.
Limitations
- Dependency on black-box LLMs: Mitigation via robust evaluation protocols and diverse datasets.
- Multi-agent safety concerns: Addressed through comprehensive safety frameworks and real-time monitoring.
References
[1] Achiam, J., Adler, S., et al., “GPT-4 Technical Report,” arXiv:2303.08774, 2023.
[2] Chang, Y., Wang, X., et al., “A Survey on Evaluation of Large Language Models,” ACM Transactions on Intelligent Systems and Technology, 2024.
[3] Wang, L., Ma, C., et al., “A Survey on Large Language Model Based Autonomous Agents,” Frontiers of Computer Science, 2024.
(And more references as per the original document…)
This Markdown document was converted by ChatGPT from the original proposal document.