Biomedical Knowledge Integration and Editing for Large Pretrained Language Models
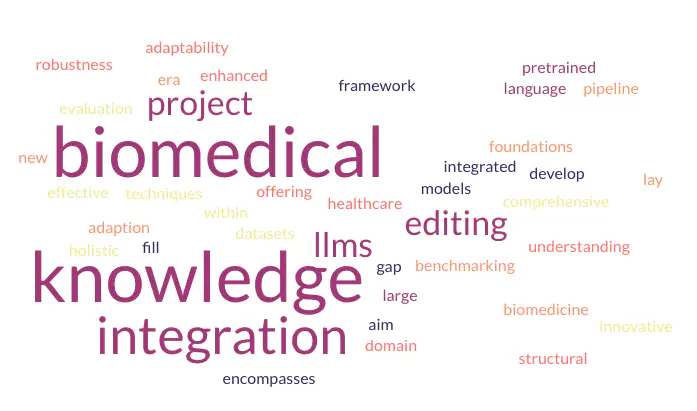
Biomedical Knowledge Integration and Editing for Large Pretrained Language Models
This proposal was originally submitted to the EPSRC NIA but was not selected for funding. However, we remain committed to bringing this project to life. Check out my Google Scholar for my latest research related to this project.
1. Vision
Large Language Models (LLMs) are significantly reshaping the landscape across various global domains, including the field of biomedicine. Within biomedicine, LLMs have demonstrated their potential in various biomedical natural language processing (BioNLP) tasks and applications—ranging from question answering and medical dialogue generation to medical education and clinical practice [8,11]—by leveraging extensive pretraining on textual data. Concurrently, biomedical knowledge graphs serve as distinctive repositories, encapsulating complex interconnections among key biomedical elements like proteins, genes, drugs, and diseases. These interactions go beyond mere textual representation, encompassing a wide array of biological attributes, including molecular functions, phenotyping, protein functional roles, and disease relationships [2]. Leveraging this structural knowledge is vital, as it underpins numerous biomedical applications, ranging from drug target identification and drug repurposing to protein docking, gene–disease association prediction, ligand–receptor interaction prediction, and disease module identification [6,9].
Despite their potentials, current LLMs face challenges in effectively assimilating and fully harnessing biomedical knowledge, underscoring the need for an integrated, comprehensive collection of biomedical knowledge graphs. Given the swift progression of biomedical research, knowledge within LLMs can quickly become obsolete, making it imperative to have efficient integration and editing mechanisms that update models cost-effectively without the need for exhaustive retraining, thus ensuring timely relevance and accuracy. Moreover, the absence of standardized techniques for infusing and editing biomedical knowledge into LLMs, along with a lack of a holistic evaluation and benchmarking framework, further highlights the aim of our project.
In this project, we aim to fill this gap and develop a framework that encompasses integrated and comprehensive biomedical knowledge datasets, innovative techniques for effective integration and editing of biomedical knowledge within LLMs, and a holistic evaluation and benchmarking pipeline. This project will lay the foundations for a new era of LLMs’ adaption in biomedicine and healthcare, offering enhanced domain understanding, adaptability, and robustness through structural biomedical knowledge integration.
1.1 Importance and Excellent Quality
Although LLMs have already demonstrated transformative capabilities across diverse domains, the integration of structured biomedical knowledge offers an unparalleled opportunity to elevate the precision, applicability, and reliability of LLM-based solutions within the context of biomedicine and healthcare. Healthcare tasks, such as patient care encompassing disease treatment, and biomedical research involving the scientific exploration of novel therapies, demand specialized expertise that is both limited and costly to acquire [1]. In light of the escalating global demand for safe and effective medications to cater to burgeoning aging populations and advancing insights into disease burdens [5], the urgency for innovation is evident. Presently, the journey from novel drug discovery to market availability spans a substantial 13–15 years, requiring an average investment of US$2–3 billion [10].
The significance of the proposed project is deeply rooted in its potential to revolutionize the field of drug discovery by encapsulating LLMs with complex interconnections among key biomedical elements. For instance, a pretrained protein language model that matches target proteins and potential drug molecules without the computationally intensive step of calculating each protein’s 3D structure from its amino acid sequence can screen more than 100 million drug-protein pairs in a single day [17]. By effectively bridging the prevailing chasm between advanced LLMs and intricate biomedical knowledge graphs, this project achieves a dual-purpose impact. It not only enriches the realm of BioNLP tasks, equipping the biomedical community with powerful tools for navigating intricate medical information, but also directly addresses the imperative of seamlessly integrating healthcare data with molecular, genomic, and disease drug insights, crucial for advancing drug discovery. This synergy yields more precise and interpretable predictions encompassing the breadth of biological systems, from genotype–phenotype associations to population-scale epidemiological dynamics [6], thus facilitating the seamless flow of biomedicine research, medical diagnosis, and drug discovery.
1.2 Beyond the Current State of the Art and Generation of New Ideas
While existing applications of LLMs have demonstrated their capabilities [8], they often rely on surface-level language understanding (such as producing hallucinated information) and lack the ability to comprehensively tap into the intricate relationships embedded within biological attributes. Thus, advances in LLMs and biomedical knowledge underscore the urgency and relevance of investigating innovative techniques for effectively integrating and editing biomedical knowledge within LLMs. While prior research demonstrated the feasibility of probing and integrating biomedical knowledge into smaller language models [TR6-7], these efforts primarily focused on textual entities and small models. In recent studies [13,15], attempts have been made to integrate textual data with protein sequence information, although their validation scope remains limited across a wider range of biomedical tasks.
Our project addresses these limitations through the development of a comprehensive framework that seamlessly integrates and edits biomedical knowledge across various entity types (e.g., the amino acids of a protein, textual name of a disease, as well as their relations) within LLMs. By subjecting our approach to rigorous validation across a wide spectrum of biomedical tasks (including BioNLP tasks such as question answering and life science prediction tasks such as drug-target prediction), we bolster both the robustness and versatility of applications while seamlessly amalgamating structural and functional insights into LLMs. Through the utilization of our extensive biomedical knowledge graphs collection, innovative integration and editing techniques, and holistic evaluation mechanisms, we stand poised to revolutionize the utilization of LLMs in biomedicine, effectively bridging the gap between linguistic comprehension and domain expertise. Moreover, the development of a standardized evaluation and benchmarking framework will ensure not only the transparency and reliability of our approach but also the foundation for a shared platform where researchers and practitioners can collaboratively enhance and validate the capabilities of their knowledge-enhanced biomedical LLMs.
1.3 Timelessness and Novelty of the Research
Amid rapidly evolving technological landscapes, our project seamlessly aligns with current trends and needs in biomedicine and natural language processing. The significance of LLMs has reached unprecedented heights, reshaping information processing across domains [11][TR2,4]. However, using AI for scientific innovation and discovery (e.g., biomedicine research and drug discovery) presents unique challenges compared with other areas of human endeavor where AI is utilized [12]. As large-scale biomedical data becomes more accessible and demand for efficient insights extraction grows, our project’s timing is opportune [2]. By bridging LLMs with biomedical knowledge, our project addresses this need, advancing AI-driven solutions for understanding, interpreting, and generating biomedical information.
Furthermore, the project’s emphasis on comprehensive knowledge integration and editing techniques is timely within the broader context of responsible AI development. Our project’s dedication to holistic evaluation and benchmarking frameworks resonates with the increasing industry focus on accountability and reproducibility in AI research and application, specifically within the realm of scientific discovery and biomedicine. By addressing prevailing trends and pressing needs, our project not only propels the forefront of biomedicine advancements but also lays the cornerstone for responsible and influential AI technologies in healthcare and beyond.
1.4 National and Global Importance and Impact
The proposed project holds substantial significance on both the national and global levels. At the national level, it holds the potential to address major challenges in AI-enhanced biomedicine, positioning the UK as a forefront leader in healthcare and biomedicine innovation. Our project aligns with and complements several others:
- EP/V029045/2: Leverages machine learning to predict drug response from limited experiments.
- EP/V052527/1: Models multi-modal genomic data as an integrated system.
- EP/W02909X/1: Uses deep graph neural networks to model cellular topology and spatial heterogeneity in large whole slide images for personalized medicine.
- EP/V050869/1: Uses machine learning techniques to support knowledge graph construction and curation, complementing our work and enabling the use of up-to-date knowledge graphs.
By bridging the gap between complex biomedical knowledge graphs and LLMs, the project could empower healthcare practitioners, researchers, and policymakers with a more comprehensive understanding of disease mechanisms, treatment options, and patient outcomes. This can lead to improved clinical decision-making, streamlined research efforts, and efficient drug discovery, ultimately contributing to the enhancement of public health and well-being within the UK.
On a global scale, by addressing the limitations of existing LLMs in effectively integrating biomedical knowledge, the proposed framework could serve as a pioneering model for knowledge incorporation in various domains. The development of standardized techniques for biomedical knowledge integration and editing, along with a holistic evaluation and benchmarking framework, could set a precedent for enhancing the accuracy, reliability, and applicability of LLMs across disciplines beyond biomedicine. Furthermore, by contributing to the evolution of LLMs as a globally applicable tool, the project may foster international collaboration, positioning the UK at the forefront of AI-driven biomedical innovation and research.
1.5 Direct and Indirect Beneficiaries
The project holds the potential to bring about transformative benefits to various stakeholders within the AI and biomedicine domains. By crafting a framework that deeply integrates extensive biomedical knowledge into LLMs, researchers gain an advanced tool, continually updated with the latest biomedical insights, aiding in tasks ranging from automated text analysis and medical literature summarization to predicting protein functions and drug-target prediction. This capability not only saves time but also enhances the accuracy and reliability of information extraction and prediction, thus facilitating more informed and effective research and healthcare practices.
Additionally, industries related to pharmaceuticals and biotechnology can capitalize on the advanced capabilities of knowledge-enhanced LLMs. Drug discovery and development processes can be streamlined by identifying potential drug targets, predicting drug interactions, and repurposing existing drugs for new applications. In comparison to past reliance on Graph Neural Networks (GNNs) in drug discovery, which primarily focused on molecular models, LLMs offer a broader scope, delving into extensive textual data and decoding the intricate tapestry of biological relationships over diverse biomedical entities. The enhanced depth and holistic capabilities of knowledge-enhanced LLMs represent a significant shift in AI’s biomedicine role, interpreting and crafting intricate textual data in ways that GNNs could not traditionally achieve. This not only reduces costs and time but also contributes to the acceleration of novel therapeutic interventions. Ultimately, the holistic project addresses the needs of a diverse range of beneficiaries, fostering advancements in biomedical research, healthcare delivery, and industry practices.
2. Approach
The goal of this project is to enhance LLMs with biomedical knowledge graphs and to develop efficient and effective techniques for editing and fine-tuning these models with biomedical knowledge graphs. This enhancement aims to improve their accuracy, timeliness, and applicability in the biomedical domain. This project takes the first steps toward this goal with the following objectives.
2.1 Objectives
- O1. Data Collection: Collect and curate diverse biomedical knowledge graph datasets, including medical disease, gene, drug, protein, and molecular databases, for integrating into LLMs.
- O2. Knowledge Integration Pipeline: Design and implement a pipeline with algorithms and pre-processing methods for effectively integrating structured biomedical data (e.g., ontologies, knowledge graphs) into the pretraining and fine-tuning processes of biomedical LLMs.
- O3. Biomedical Knowledge Editing Techniques: Develop models that locate the knowledge neurons in LLMs and then apply modifications to them to edit and fine-tune the knowledge LLMs for specific biomedical tasks and applications.
- O4. Evaluation and Benchmarking: Design rigorous evaluation protocols to benchmark the modified LLMs’ performance on various biomedical tasks. Develop automated testing procedures and metrics to assess model accuracy, timeliness, and applicability to biomedical applications.
Feasibility and Preliminary Results: This project will last 36 months. Progress has already been made toward the objectives by the PI and collaborators. The team has experience in collecting biomedical data [TR3,6-7,9], laying the foundation for O1. Prior works [TR1-2,4,7,8-11] demonstrate expertise in knowledge integration and fine-tuning, supporting O2 and O3. Collaborations with N. Collier (University of Cambridge) and E. Shareghi (Monash University) [TR6-7,9] highlight the team’s commitment to rigorous evaluation (O4).
2.2 Research Program and Methodology
WP1: Dataset Collection (M1–M10)
This work package focuses on collecting and curating biomedical knowledge graphs and evaluation datasets, spanning textual, genetic, protein, drug, and molecular domains. Key resources include:
- Knowledge Graphs: UMLS, SNOMED, PrimeKG, Reactome, DisGeNET, DrugBank.
- Evaluation Datasets:
- BioNLP tasks: BioNLP-OST, BLURB (for NER, relation extraction).
- Life science prediction tasks: TorchDrug, TDC, xTrimo (entity generation, multi-instance prediction).
Outcome 1: Curated biomedical knowledge graphs and benchmark datasets for integration and evaluation, laying the groundwork for tasks like NER, relation extraction, and life science predictions.
WP2: Biomedical Knowledge Integration and Editing Techniques (M9–M28)
Building on prior studies [13,15,16] and PI’s work [TR6-7], this WP develops methods to integrate structural biomedical knowledge into LLMs. Strategies include:
- Partitioning knowledge graphs and parameter-efficient fine-tuning.
- Integrating structural knowledge into generative models for concurrent comprehension of entities (e.g., proteins, molecules).
- Enhancing knowledge editing techniques [3,14] for biomedical LLMs, leveraging layer-wise probing [TR6].
Outcome 2: Advanced integration and editing methodologies to enhance LLMs’ contextual understanding and adaptability to evolving biomedical knowledge.
WP3: Evaluation and Benchmarking Framework (M4–M30)
This WP establishes a comprehensive evaluation strategy using datasets from WP1. Key components:
- Benchmarking across BioNLP (e.g., BioNLP-OST) and life science prediction tasks (e.g., TorchDrug).
- Developing an interactive leaderboard and evaluation pipeline for real-time assessment.
Outcome 3: A holistic evaluation system with a leaderboard to validate LLM performance and foster continual refinement in biomedical language modelling.
WP4: Dissemination, Exploitation, and Impact (M7–13, M28–M36)
Aligned with EPSRC’s AREA framework, dissemination strategies include:
- Open-access publications (ArXiv, Zenodo).
- Publicly available models (GitHub, HuggingFace) and benchmark platforms.
- Workshops and social media engagement (Facebook, Twitter).
- Collaboration with BioMed X Institute for case studies.
Outcome 4: Ethical dissemination ensuring broad access to resources, fostering collaboration, and maximizing societal impact in AI and biomedicine.
This Markdown document was converted by ChatGPT from the original proposal document.