BioCaster
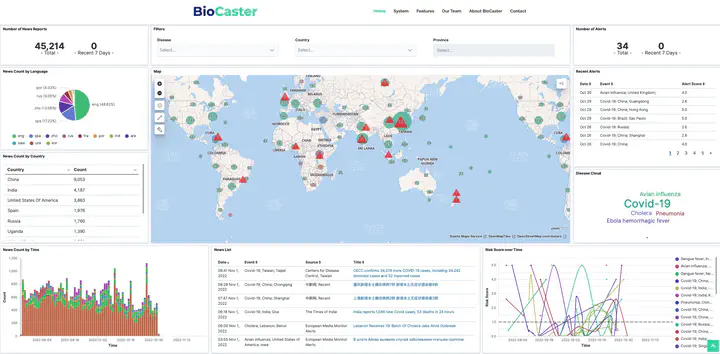
BioCaster is a research project aimed at providing advanced search and analysis of Internet news and research literature for public health workers, clinicians and researchers interested in communicable diseases.
The emergence of disease outbreaks is of the greatest importance to the international community. Making a rapid response crucially depends on having timely evidence, yet traditional bio-surveillance using human networks is often unavailable in real-time, patchy in geographic coverage, and tuned to specific diseases. Digital disease surveillance (DDS) using Web-based news and social media data aims to overcome some of these limitations. Real-time DDS was pioneered in the early 2000s by the Canadian GPHIN system, which detected the first SARS evidence and in more recent times has been joined by other systems such as BioCaster.
BioCaster is a fully automated real-time media monitoring system based on Natural Language Processing (NLP) technology. The system was first launched in 2006 as a research prototype by Dr Nigel Collier at the National Institute of Informatics in Japan and ran until 2012 with funding support from multiple sources include the Japan Society for the Promotion of Science. With funding from the Canada-UK Artificial Intelligence Initiative, Principle Investigators from Cambridge University and McGill University have partnered to upgrade and re-launched BioCaster as part of the EPI-AI project. EPI-AI is a team of epidemiologists, computer scientists, social scientists and computational biologists working together to improve early warning for public health. Both PI’s are currently members of the WHO’s Epidemic Intelligence from Open Sources (EIOS) initiative.
Early detection and tracking of infectious disease outbreaks involves having access to information from a variety of sources. Increasingly this means monitoring many thousands of Internet news feeds simultaneously. However three difficulties exist in finding information using traditional search methods: firstly the massive volume of dynamically changing unstructured news data makes it extremely difficult for governments and public health workers to obtain a clear picture of the outbreak. Secondly, the initial reports of an outbreak are contained in only a few news articles which will usually be overlooked using simple keyword indexing methods. Thirdly, the initial reports of an infectious disease will usually be reported in local none-English news media. In order to capture outbreak information in the most timely manner it is therefore crucial for computer systems to have an understanding of several languages. As part of the EPI-AI project we have partnered with SDL (now part of RWS) to use their Machine Translation Edge technology to overcome the language barrier in 10 languages: Arabic, Chinese, French, Indonesian, Farsi, Korean, Portuguese, Spanish, Russian, and Swahili.
The second generation of BioCaster has two major components: a web/database server (built on Elasticsearch and Kibana) and a backend cluster computer (Rocks) equipped with hybrid symbolic-neural NLP technology which continuously scans hundreds of RSS newsfeeds from local and national news providers. Since the NLP system has a detailed knowledge about the important concepts such as diseases, pathogens, phenotypes, people, places, drugs etc. this allows us to semantically index relevant parts of news articles, enabling users to have quicker and highly precise access to information. The knowledge we use comes from annotated text collections (e.g. the PheneBank corpus and the COMETA corpus), gazetteer lists of nomenclature and the BioCaster ontology, all of which are currently under development. We are making the new BioCaster system available for public access and feedback in the hope that it will be useful to those interested in the field. Software resources are also expected to be released as the project progresses. Supplementary information will be published in international conferences and journals.